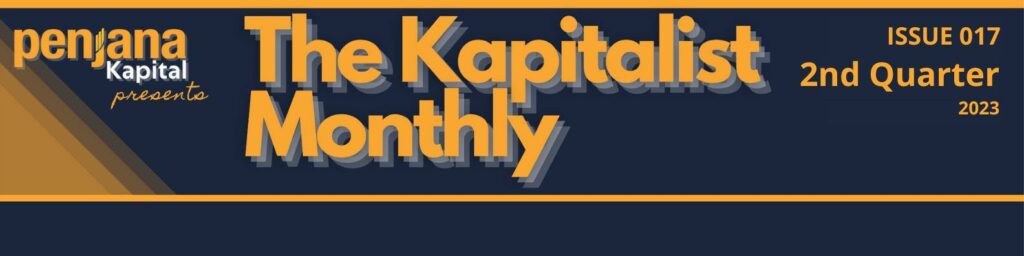
Dear Readers,
Welcome to another edition of Penjana Kapital’s Flagship Kapitalist Newsletter. In this new format, we will publish a research-based newsletter on a quarterly basis featuring a deep dive into tech’s biggest topics globally.
We at Penjana Kapital are pleased to release a full research report on Artificial Intelligence (AI) and its impact on the efficiency mechanisms on various key sectors such as Financial Technology (Fintech), Manufacturing and Internet of Things. Judging from the recent key headlines on news sites such as Bloomberg and long conversational threads on Twitter, it is obvious AI has experienced significant growth and advancement in recent years, transforming various industries and becoming an integral part of our daily lives.
Whether we realise it or not, we experience AI everyday – AI has become integrated into our daily lives through voice assistants like Siri and Alexa, recommendation systems on streaming platforms, smart home devices, virtual reality, and augmented reality applications. From personalised content delivery to smart home automation, AI technologies have seamlessly become part of our routines, enhancing convenience and efficiency. On the Business to Business (B2B) side, many industries, including healthcare, finance, manufacturing, retail, transportation, and entertainment, have embraced AI solutions. Organisations are leveraging AI to streamline processes, enhance productivity, improve customer experiences, and gain competitive advantages. From virtual assistants and chatbots to personalised recommendations and autonomous vehicles, AI has become a cornerstone of innovation across sectors.
AI has attracted substantial investment from both private and public sectors. Venture capital funding for AI startups has soared, reflecting the confidence in the technology’s potential. Additionally, governments and organisations worldwide have allocated significant budgets for AI research and development, further fuelling its growth. Venture capital (VC) and AI are two interconnected realms that have witnessed significant growth and collaboration in recent years. Microsoft recently made a “multiyear, multibillion-dollar” investment in OpenAI, the AI lab behind the experimental online chatbot ChatGPT. Microsoft had already invested more than $3 billion in OpenAI, and the new deal is a clear indication of the importance of OpenAI’s technology to the future of Microsoft and its competition with other big tech companies like Google, Meta and Apple.
The intersection of VC and AI has created a thriving ecosystem where investors recognise the immense potential of AI technologies and actively seek opportunities to fund and support AI-driven startups. Startups leveraging AI technologies such as Machine Learning (ML), natural language processing, computer vision, and robotics attract the attention of venture capitalists due to their potential for disruption and high growth prospects. AI algorithms, particularly deep learning models, often require substantial computational resources, hindering their scalability and wider adoption. Developing efficient algorithms that can run on low-power devices and optimising resource utilisation are essential for democratising AI technology.
- Data Analysis and Insights: AI excels at processing and analysing vast amounts of data quickly and accurately. Businesses can leverage AI to extract valuable insights, identify patterns, and make data-driven decisions. AI-powered analytics enable businesses to gain a deeper understanding of customer behaviour, market trends, operational efficiency, and optimise strategies accordingly.
- Automation and Efficiency: AI can automate repetitive and time-consuming tasks, freeing up human resources to focus on more complex and strategic activities. From chatbots and virtual assistants handling customer inquiries to robotic process automation (RPA) streamlining back-office operations, AI-driven automation can significantly enhance efficiency, reduce costs, and improve productivity.
- Personalisation and Customer Experience: AI enables businesses to deliver personalised experiences to their customers. By leveraging ML algorithms, businesses can analyse customer data, preferences, and behaviour to provide tailored recommendations, personalised marketing campaigns, and customised products or services. This level of personalisation enhances customer satisfaction, engagement, and loyalty.
- Fraud Detection and Risk Management:AI can identify and flag anomalous patterns or fraudulent activities by analysing large datasets in real-time. Financial institutions can employ AI algorithms to detect fraudulent transactions, monitor cybersecurity threats, and mitigate risks. AI-powered fraud detection helps businesses protect their assets, customer data, and maintain the integrity of their operations.
- Natural Language Processing and Conversational Interfaces:AI technologies, such as natural language processing (NLP), enable businesses to understand and respond to human language. Chatbots and virtual assistants can handle customer inquiries, provide support, and offer personalised recommendations. NLP capabilities also extend to sentiment analysis, social media monitoring, and content curation, allowing businesses to gather valuable insights from unstructured data.
- Product Development and Innovation:AI can accelerate product development cycles and fuel innovation. Businesses can utilise AI algorithms to analyse market trends, consumer preferences, and competitor landscapes to inform their product design and development processes. AI-driven simulations and predictive modelling can also aid in optimising product performance and reducing time-to-market.
Decision Support Systems: AI-powered decision support systems can assist businesses in complex decision-making processes. By analysing relevant data, historical trends, and market conditions, AI algorithms can provide recommendations or insights that aid executives in strategic planning, risk assessment, and resource allocation. This enables businesses to make more informed and data-driven decisions.
In this issue, we also hope to address some of problem statements faced by AI. Our analysts have prepared detailed reports of 4 subsectors in this issue addressing these problems.
- Data Quality and Availability: The performance of AI algorithms heavily relies on the quality and availability of data. However, obtaining labelled and diverse datasets can be challenging, especially in domains where data collection is expensive or restricted. The problem of data scarcity and bias remains a significant obstacle to the accurate and fair functioning of AI systems.
- Human-AI Collaboration: AI should be viewed as a complement to human capabilities rather than a substitute. The challenge lies in creating interfaces and frameworks that facilitate effective collaboration between humans and AI systems, enabling them to work together seamlessly and leverage their respective strengths.
- Robustness and Security: AI systems are vulnerable to adversarial attacks, where malicious actors manipulate inputs to deceive or exploit their weaknesses. Ensuring the robustness and security of AI models is critical to prevent manipulation, unauthorised access, or potential harm caused by compromised systems.
- Legal and Regulatory Frameworks: The rapid advancement of AI technology has outpaced the development of comprehensive legal and regulatory frameworks. Establishing policies that govern the responsible development, deployment, and usage of AI systems is crucial to address potential risks and ensure ethical practices.
It is more than a year since Russia’s invasion of Ukraine and the grinding conflict shows no sign of abating. It has however unveiled a prospect of greater clash. Ukraine is now the centre of America’s (and its allies) competition with China (and its allies). There are gathering storm clouds of possible civilisational conflict between Pax Americana and Pax Chinica. Even though the proverbial thunderstorm has not yet burst forth however we do face the prospect of the grey chilling and unceasing drizzle of another ‘Cold War’ between these two superpowers. There is no playbook for managing this tension, and the rivalry has extended to technology arms race. AI is a strategic weapon whose development and deployment play a critical role in this tech battlefield. It is the next frontier in the race where the possible winner may take it all and claim victory.
Only these two superpowers – America and China – have AI in their armoury. It is probably best to think of a ‘Cold War’ framework and to see this as the latest feature of the arms race that characterises cold wars. Nuclear arms race had defined the First Cold War whilst the AI arms race will determine the New Cold War. Both wars are unnervingly similar but there is a variation. One obvious difference between the older deadly nuclear technology and AI is that most research and discoveries on AI are being led by the private sector. Private sector actors are developing the next generation of technology, in marked contrast to mid twentieth century innovation in which The Manhattan Project was a government enterprise. The arms race in AI now is really between a handful of companies though it is no secret they are either backed by these superpowers or are subservient.
Nuclear is one form of technological innovations that might lead to the destruction of homo sapiens. A view shared by a group of chief executives and scientists from technology companies, AI’s risk to humanity is on par with nuclear war.
“Mitigating the risk of extinction from AI should be a global priority alongside other societal-scale risks such as pandemic and nuclear war”.
Those who argue that AI technology is as dangerous as a nuclear weapon believe essentially if we create an intelligence so superior to human intelligence, it will wipe us out. Eliezer Yudhowsky wrote for Time magazine, “The most likely result of building a superhumanly smart Artificial Intelligence under anything remotely like the current circumstances is that literally everyone on Earth will die”. It is a bit like Liu Cixin’s book The Dark Forest – the sequel to The Three-Body Problem novel. Liu avows that if there is any superior intelligence in the Universe, and it discovers us, it will immediately eradicate us (just in case we might eradicate it).
But I attempt to shake things up and make this a critical discourse.
These people are smarter than me and when they point out there is non-trivial risk that AI is out to kill us, I do listen intently. It is the Skynet scenario from The Terminator movie. Though I watched and loved the franchise, I do however admit I am not entirely a subscriber to that view. I have a somewhat different take on the threat posed by AI’s Large Language Models (LLM). If one would think about the potential applications of LLM in politics, this will be an even more potent tool for polarisation and the spreading of disinformation than social networks and social media were back in the previous decade. It is not strange for most to be very uneasy about the speed with which LLM are being rolled out.
The proponents of unrestrained development of AI like Reid Hoffman do not seem to address that issue, and they should because it is pretty much the same people that give us the social networks online. I do not think any of them really can claim today that social media did not have a major political downside. So, I do hope, in the spirit of freedom of speech in politics and of freedom of knowledge in open architecture, we are discussing more urgently the potential use and implications of AI in politics and other aspects of our society. It is incredibly a powerful tool that can be used to cause even more pandemonium in our society than social media has caused so far. I am not denying that AI is useful.
In fact, by acknowledging its potential threat, we simultaneously admit that it is hugely powerful. For instance, AI is extremely advantageous for medical scientific research but there are other ways in which AI in the realm of biotech could be dangerous. Just in the same way AI’s programme AlphaZero mastered chess in a different way from us, we should also approach this discussion in a different way from programmed script – this is not all too simple, it is complex. We should explore domains and sectors that are most likely to be revolutionised by it and anticipate possible downsides. This is complex but our conscience is clear. Our brilliant mind came out with this technology that has the power to disrupt (and possibly destroy) us, and it is up to us at this stage to figure out future path and set guardrails the way forward.
“America and China might be entering a New Cold War, and both are in the race for technology supremacy particularly in the realm of AI and computing. But the ‘real’ cold war is between us and the future. The point of a ‘Cold War’ is that it is not really a clash. A cold war is a peace that is no peace, as in the words of George Orwell. We’d only live in peace for as long as we don’t stop learning and thinking about tomorrow.”
How Far Along Are We?
When talking about the consumer automotive industry, there are predominantly two areas that are growing aggressively: (i) Electric Vehicles (EV) and (ii) Autonomous Driving (AD). These two areas’ growth over the past few years have been nothing short of tremendous, especially as AI hardware and software capabilities saw similar growth trajectories. According to McKinsey, by 2035, AD technology alone could create $300 billion to $400 billion in revenue, as the consumer interest and AD technology matures. Companies will need to adapt to evolving consumer demands and technology and understand the needs of consumers before cementing their position in this space.
AD technology has in many ways benefited from the developments in AI, such as:
Improved Perception and Decision-making
AI is helping autonomous vehicles to better understand their surroundings and make more informed decisions. For example, AI-powered sensors can now detect objects that are difficult for human drivers to see, such as small animals or pedestrians. AI is also being used to develop more sophisticated algorithms that can better predict the behaviour of other road users. Waymo, Alphabet’s AD subsidiary, has made progress in developing AD software that can identify objects (e.g., pedestrians, construction obstacles, etc) and read traffic signs (e.g., speed limit signs, stop signs, traffic lights, etc.). Waymo claims to be one of the global leaders in AD, and that their Driver AI is safer than a human driver (this remain disputed as the test was done in highly controlled scenarios).
These capabilities are all thanks to a combination of ML, NLP and computer vision, which together aim to replicate a human being manning the vehicle.
Increased safety
AI is helping to make driving safer by reducing the number of human errors. According to Professor Robert W. Peterson of the Center for Insurance Law and Regulation at Santa Clara University School of Law, over 90% of accidents today are caused by driver error. Thus, AI-powered vehicles, which are not susceptible to fatigue, distraction, or intoxication can potentially mitigate this risk.Nonetheless, getting to Level 5 Autonomous Vehicles that can almost completely eliminate road accidents will take huge amounts of funding and R&D, if we even succeed. Case in point: even after almost a decade of releasing the first version of Autopilot, Tesla’s crashes accounted for nearly 70% of the 392 reported between July 2021 and May 2022 (It is important to note, however, that statistics pertaining to AD-related crashes are still sparse).
Lower costs
AI is helping to lower the cost of autonomous driving by making it possible to develop more efficient and reliable systems. For example, AI can be used to optimise the routing of autonomous vehicles by relying on advanced ML capabilities combined with real-time traffic data, which can potentially help to reduce fuel consumption and operating costs.
Enhanced User Experience
AI is also being used to enhance the user experience of autonomous vehicles. For example, AI can be used to provide passengers with entertainment and information, as well as to monitor their health and well-being. Waymo’s robo-taxi passengers can easily tune in to their favourite shows or listen to their favourite tunes and relax at the back of the car.
The Road to Full AD is a Long, Costly Journey.
AD’s massive improvements over the past years have caught the attention of investors. As a result, investors are seeking to get exposure to companies that are in the EV and AD space – companies striving to disrupt the automotive industry (Year to date, the Global X Autonomous & Electric Vehicles ETF (DRIV) is up 25.67%, beating the SPDR S&P 500 ETF Trust (SPY) which is up by 13.28% over the same period). DRIV invests in companies that are involved in the development and manufacturing of software and hardware for autonomous vehicles, and companies that produce electric vehicles and their components, such as lithium and cobalt. DRIV’s holdings include NVIDIA, Apple, Tesla, Alphabet, Toyota and Intel.
YTD Chart of the Global X Autonomous & Electric Vehicles ETF (DRIV) and the SPDR S&P 500 ETF Trust (SPY)
Source: Bloomberg
Source: PitchBook
Tesla, the darling of the global EV and AD space, has always commanded massive valuation premiums over its peers.
Source: Bloomberg
What’s the Hold Up?
While the opportunities that come with Level 5 AD sounds extremely exciting (the average Malaysian spends 44 hours in traffic in a month – imagine the things we can achieve during this time), there are several reasons why autonomous vehicles are still not mainstream on top of what has been mentioned above. These include:
- Technical challenges. The technology for autonomous driving is still in its early stages of development. There are many challenges that need to be solved before autonomous vehicles can be fully safe and reliable. For example, autonomous vehicles need to be able to handle a wide variety of road conditions and traffic situations. They also need to be able to make quick and accurate decisions in emergency situations. AI struggles with uncertainty, and there is plenty of unexpected things that can happen while driving.
- Regulatory hurdles. Governments around the world are still working out the regulations for autonomous vehicles. This is a complex process, and there is no guarantee that all governments will agree on the same set of rules.
- Public acceptance. Some people are still not comfortable with the idea of autonomous vehicles. They worry about the safety of these vehicles, and they may not be willing to give up control of their own driving. 68% of drivers surveyed by the American Automobile Association in January 2023 are afraid of riding in a self-driving vehicle. That is up from 55% in 2022 and is the largest annual increase since 2020.
- Lack of data. Autonomous vehicles need a lot of data to train their algorithms. This data includes information about road conditions, traffic patterns, and the behaviour of other drivers. There is not yet enough of this data available to train autonomous vehicles to be fully safe and reliable. Waymo, for instance, currently only operates a few hundred self-driving cars in each of its Waymo One service areas, limited to Phoenix and San Francisco, with testing taking place in Austin and Los Angeles. Autonomous vehicles are still operating only in controlled areas.
- Ethical dilemmas. This could maybe be one of the hardest issues to solve when it comes to training the AI for autonomous vehicles. Driving involves tonnes of variables and scenarios that require a human’s judgement, which AI clearly lacks. For example, what should an autonomous vehicle do if it has to choose between hitting a pedestrian or swerving into another car? There is no easy answer to this conundrum, and it is one that will need to be addressed.
Overall, we are still a long way from mass-adoption of Level 5 AD. However, advancements in the technology are moving rapidly, thanks to the recent growing interest towards AI. ML will continuously develop AI that will then at some point be as capable as a human brain. Add to this the hardware improvements in chips and semiconductors, one could dream that the utopian future of transportation-as-a-service could maybe, just maybe, be a case of When, not If.
The global healthcare industry is vast and diverse, encompassing a wide range of sectors such as hospitals, clinics, pharmaceuticals, medical devices, telemedicine, and health insurance. According to Business Wire, the global healthcare industry was valued at over $8 trillion in 2021 and is projected to experience robust growth in the coming years. The increasing demand for quality healthcare services, aging populations, and the prevalence of chronic diseases are some of the key drivers fuelling this growth. AI has the potential to address these challenges by improving the efficiency and effectiveness of healthcare delivery.
According to Pitchbook, there were 70 VC rounds completed, totalling $1.3 billion in the first quarter of 2023. While this showed improvement compared to the previous quarter, it still fell short of the pace set between 2020 and 2021. In 2021, VC invested $12.8 billion in AI healthcare start-ups. Additionally, 27 private equity deals were finalised during the quarter, which remained relatively stable compared to the previous quarter. Despite the somewhat uninspiring deal flow, the pressing need for advancements in healthcare delivery, reimbursement, and operations continues to generate interest in healthcare IT.
AI is revolutionising healthcare
Source: Global AI in Healthcare Forecast, Markets and Markets (2019)
The Healthcare Information and Management Systems Society Conference, held from April 17 to 21, 2023, served as a significant indicator. The event emphasised the potential of generative AI and interoperability solutions, both of which have the potential to be transformative but are currently limited in their applications.
The use of AI in healthcare is still in its early stages, but it has the potential to transform the way we deliver care. AI can analyse vast amounts of clinical data quickly and accurately, identifying patterns and trends that would be overlooked by human experts. AI improves the accuracy of diagnoses, the effectiveness of treatments, and the efficiency of care delivery.
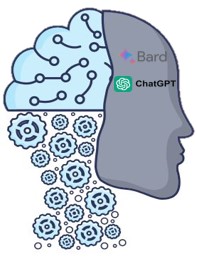
The next generation of LLMs, such as GPT-4, will transform healthcare, starting with non-medical applications like patient engagement and EHR summaries. Later, they will be used for medical applications like symptom triage and prior authorisation reviews. Eventually, they will be able to make autonomous medical decisions. This progression will take 5-10 years or more, and it will be important to ensure transparency and explicability.
There are a number of areas where AI is disrupting the healthcare industry:
1. Diagnosis: AI is revolutionising medical diagnostics by enabling faster and more accurate analysis of medical images, such as X-rays, CT scans, and MRIs. ML algorithms can quickly identify patterns and anomalies, aiding in the early detection of diseases like cancer, cardiovascular conditions, and neurological disorders. AI-powered diagnostic tools reduce human error, improve efficiency, and enhance patient outcomes.
Melanoma, a malignant tumour responsible for most skin cancer-related deaths worldwide, poses a challenge in early detection due to the large number of pigmented lesions requiring evaluation. Researchers from MIT and other institutions have developed an AI pipeline that uses deep convolutional neural networks (DCNNs) to analyse suspicious pigmented lesions (SPLs) using wide-field photography from smartphones and personal cameras. By taking wide-field photographs and applying DCNNs, the programme can quickly and effectively identify early-stage melanoma, improving prognosis and reducing treatment costs.
The system achieved over 90.3% sensitivity in distinguishing SPLs from nonsuspicious lesions and backgrounds, providing comparable accuracy to expert dermatologists. This research aims to enhance dermatological screenings in primary care settings and enable prompt treatment of melanoma.
2. Treatment: AI-powered treatments, such as robotic surgery, are being developed to enhance healthcare by improving precision, safety, and patient outcomes. These AI-powered robots offer superior precision, real-time data analysis, and the potential for personalised treatment plans. While challenges remain, integrating AI into healthcare holds promise for revolutionising medical practices.
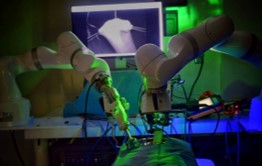
In a ground-breaking development, a robot successfully performed a laparoscopic surgery without the guidance of a surgeon’s hand. The Smart Tissue Autonomous Robot (STAR) completed the challenging task on a pig’s soft tissue, demonstrating significant progress towards fully automated robotic surgeries. The STAR automated the intricate procedure of reconnecting two ends of an intestine, surpassing human surgeons in terms of results. This advancement in autonomous robotic surgery has the potential to enhance medical efficiency, safety, and reliability.
The latest STAR model utilises advanced precision, suturing tools, 3D imaging, and ML-based tracking algorithms to adjust its surgical plan in real time. It employs a ML algorithm based on convolutional neural networks to predict tissue motion and guide suture plans. By minimising human intervention, the STAR showcases its ability to plan, adapt, and execute surgical procedures in soft tissue, marking a significant milestone in the field.
3. Drug Discover: Traditional drug discovery is a time-consuming and expensive process. AI algorithms can analyse vast amounts of biomedical data, including genomics, proteomics, and clinical trial results, to identify potential drug candidates more efficiently. AI-based algorithms also facilitate predictive modelling and simulation, enabling researchers to optimise drug properties and predict efficacy. This transformative approach accelerates the drug discovery and development process, leading to faster delivery of innovative treatments.
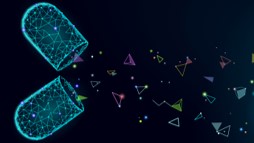
On the May 16th2023, Google Cloud launched two AI-powered tools for biotech and pharmaceutical companies to expedite drug discovery and advance precision medicine. According to CNBC, the Target and Lead Identification Suite helps predict and understand protein structure, while the Multiomics Suite aids in ingesting, storing, analysing, and sharing genomic data. These developments demonstrate Google’s commitment to the AI arms race and its effort to showcase generative AI technology.
The suites address the time-consuming and costly process of bringing new medicines to the market, providing significant time and cost savings. They have already been adopted by companies like Pfizer, Cerevel Therapeutics, and Colossal Biosciences. The Target and Lead Identification Suite streamlines the identification of biological targets for drug development, utilising AlphaFold2 to predict protein structures accurately. The Multiomics Suite supports genomic data analysis, helping companies manage large amounts of data and focus on scientific discoveries. The suites have received positive feedback from industry professionals, offering substantial time and cost savings, and enabling innovative research.
4. Healthcare Administration: AI is streamlining administrative tasks in healthcare, such as medical coding, billing, and record-keeping. Natural Language Processing (NLP) algorithms can extract relevant information from medical documents and automate coding processes, reducing errors and improving billing accuracy. AI-powered systems also enable intelligent scheduling, resource optimisation, and inventory management, leading to cost savings and operational efficiencies.
“Advances in unsupervised Machine Learning and natural language processing are now enabling intelligent data capture software to automatically learn how to identify, classify and extract patient-related information from documentation and then index those documents so they can be incorporated into patient records in both clinical and administrative applications,”
Source: In 2020, AI will aid administrators and CISOs will refresh internal security, HealthcaeITNews (2020)
5. Personalised Medicine: AI is driving the shift towards personalised medicine by leveraging patient-specific data to tailor treatment plans. ML algorithms analyse genetic profiles, patient history, and real-time health data to predict disease susceptibility, optimise drug dosages, and identify the most effective treatment options for individual patients. This approach enhances treatment outcomes, reduces adverse effects, and improves patient satisfaction.
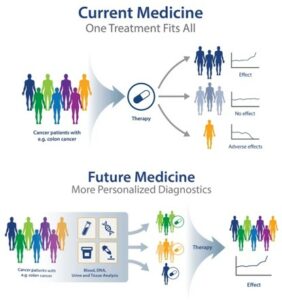
AI-powered personalised medicine has the potential to revolutionise healthcare by providing more effective treatment for common and rare diseases. By using individual health profiles rather than generic criteria like age and sex, clinicians can optimise medication timing and dosage, leading to earlier diagnosis, prevention, and improved treatment outcomes. This approach is particularly significant for addressing the gender bias in medical research, which has traditionally excluded women and neglected to analyse results by sex. Personalised medicine, by considering an individual’s gene structure, can eliminate such biases and tailor treatments specifically to each patient, ensuring better health outcomes and efficient resource utilisation.
6. Virtual Assistants and Telemedicine: AI-powered virtual assistants, chatbots, and voice recognition systems are increasingly being integrated into healthcare systems to enhance patient engagement and support remote consultations. These intelligent assistants can provide personalised healthcare recommendations, answer basic medical queries, schedule appointments, and monitor patient progress. Telemedicine platforms leverage AI algorithms to facilitate remote diagnosis, reducing the need for in-person visits and increasing access to healthcare services, particularly in underserved areas.
Source: How Healthcare Virtual Assistants are Transforming Care Continuum By Peiru Teo, KeyReply (2023)
AI-powered healthcare virtual assistants efficiently handle simple tasks, allowing medical professionals to focus on more complex responsibilities. These virtual assistants pose straightforward questions about symptoms and provide solutions based on user inputs, such as rest, scheduling appointments, or seeking emergency care. While still in the early stages, the virtual assistant market in healthcare is projected to significantly expand by 2029, with a compound annual growth rate of 26.29% between 2019-2029, according to a report on the Global Virtual Assistants in Healthcare Market.
The potential benefits of AI in healthcare are significant. AI has the potential to improve the quality of care, reduce costs, and improve patient outcomes. However, there are also a number of challenges that need to be addressed before AI can be fully realised in healthcare.
These challenges include:
1. Large datasets of high-quality data: AI algorithms need to be trained on large datasets of data in order to learn to make accurate predictions. However, healthcare data is often siloed and difficult to access. Another challenge is the need to ensure the privacy and security of patient data.
2. Data privacy and security: AI in healthcare requires access to large amounts of sensitive patient data. The abundance of personal information, such as medical records, diagnostic images, and genetic data, involved in AI-driven healthcare systems raises valid apprehensions about unauthorised access and potential breaches. This raises concerns about data privacy and security.
3. Lack of standards: The lack of standards in the development and use of AI in healthcare poses significant challenges and impedes the widespread adoption of AI-powered solutions within the industry. This absence of clear guidelines and frameworks hampers the ability of healthcare providers to effectively integrate AI technologies into their practices.
4. Meeting regulatory requirements: AI in healthcare must meet regulatory requirements to ensure the ethical use of AI systems and prioritise patient safety. Compliance with regulations is not just a recommendation but an essential aspect of implementing AI in healthcare settings. By adhering to these regulatory frameworks, healthcare organisations can establish a solid foundation for responsible AI implementation and mitigate potential risks associated with the technology.
5. Clinician acceptance: Some clinicians are reluctant to adopt AI-powered solutions. This is due to concerns about the accuracy and reliability of AI-powered tools. Physicians need to feel confident that the AI system is providing reliable advice and will not lead them astray. This means that transparency is essential – physicians should have insight into how the AI system is making decisions so they can be sure it is using valid, up-to-date medical research.
Despite these challenges, the future of AI in healthcare looks promising. Continued advancements in AI algorithms, increased adoption of electronic health records, and collaborations between technology companies, healthcare providers, and researchers will drive further disruption. AI has the potential to transform healthcare delivery, improve patient outcomes, and make healthcare services more accessible and cost-effective.
How AI is Revolutionising the Lending Industry
The beginnings of the financial lending industry trace back to ancient civilisation, mostly through informal mediums between individuals and wealthy merchants. Historically, the Romans stored deposited monies and treasures below religious temples before banking activities, including money changers, taking deposits and lending out money emerged. Through the development of trade and commerce, the need for more lending establishments arose, leading to the initial resemblance of banking institutions.
Fast forward to the present, the financial lending industry has grown quite significantly. The size of the industry is difficult to estimate precisely, as it encompasses a wide range of activities and institutions. As a gauge, the global financial services sector has reached a value of $23.3 trillion in 2021, growing at a CAGR of 3.5% since 2016. This is estimated to grow further to $33.3 trillion in 2026 and reach $45.1 trillion by 2031.
Fintech companies have disrupted the lending landscape further. Fintech firms leverage digital platforms, data analytics and algorithms to streamline lending processes, providing quicker access to credit for individuals and small businesses. They further democratise lending via innovative modes such as buy-now-pay-later, making credit lines even more accessible.
The lending industry is undergoing another major transformation thanks to the rise of AI. AI is being used to automate many aspects of the lending process, from credit scoring to fraud detection, which leads to faster, more efficient and more accurate lending decisions. The adoption of AI in the lending industry is being driven by several factors including availability of data, the growing demand for personalised lending as well as the need to reduce costs.
Lenders typically collect as many data on the borrowers to reduce defaults, from traditional financial data (e.g. credit scores, income, debt history), alternative data (e.g. social media data, mobile phone usage, online shopping) to behavioural data (e.g. timeliness of payments, utilisation of credit cards). The more data that lenders have access to, the more accurate decisions could be. Whilst data collection is important, it is also equally important to be able to be able to parse through the huge amount of available data quickly to ensure decisions can be made in a timely manner.
There are lots of ways in which AI is disrupting the lending industry including:
1. Credit scoring: AI-powered credit scoring models can extract, process and analyse large amounts of data much faster than traditional credit scoring models, allowing lenders to make more informed lending decisions in a shorter amount of time. ML models can consider a wide range of factors, including traditional credit data, alternative data sources and behavioural patterns, to make more accurate credit decisions.
2. Risk management monitoring: AI can continuously monitor borrowers’ data and market conditions, allowing lenders to proactively manage loan portfolios, identify potential defaults and optimise risk management strategies. Predictive analytics models can forecast market trends, interest rates and borrower behaviour, assisting lenders in making more informed decisions.
3. Fraud detection: AI-powered ML algorithms can detect fraudulent activities by analysing patterns and anomalies in transactions, helping lenders mitigate fraud risks.
4. Personalised lending experience: AI chatbots and virtual assistants can interact with borrowers, providing personalised and timely responses to inquiries, loan applications and support requests. Natural language processing also enables AI systems to understand and interpret customer communications, improving customer service and satisfaction.
Source: https://www.datavisor.com/
Source: https://www.haptik.ai/resources/case-study/kissht
5. Loan processing: AI technologies automate loan processing tasks, including document verification, data extraction, and loan document analysis. This reduces processing time and enables faster loan disbursements.
6. Alternative Lending: AI has facilitated the rise of alternative lending platforms, such as peer-to-peer lending and crowdfunding. These platforms leverage AI algorithms to match borrowers with lenders based on specific criteria, enabling individuals and small businesses to access credit outside of traditional banking channels.
A large amount of data bank could be a double-edged sword; hence lenders should manage the data responsibly. Consideration should be taken by lenders about how they collect and use data to ensure that it is done in a lawful manner as well as also protecting the privacy of their customers by incorporating robust cybersecurity practices. In training the AI models, it is important also to ensure the completeness, accuracy as well as ethical considerations are placed to avoid unintended consequences.
Below are some risks involved when using AI in lending:
1. Biased lending: AI systems heavily rely on historical data to make lending decisions. If the training data is biased or contains discriminatory patterns, AI algorithms can perpetuate these biases leading to unfair lending practices. Relying solely on AI-driven lending decisions may exclude certain individuals or groups who don’t fit traditional lending profiles. This could hinder financial inclusion and overlook important contextual factors that AI algorithms may not consider.
2. Model robustness: AI models require continuous monitoring and maintenance to ensure they remain accurate and effective. Inadequate model updates or failing to adapt to evolving market conditions may lead to unreliable lending decisions.
3. Security and privacy breaches: AI systems handle vast amounts of sensitive financial data making them attractive targets for cyberattacks. If not adequately protected, these systems can lead to data breaches, financial fraud or identity theft.
4. Lack of transparency: AI algorithms can be complex making it difficult to understand how decisions are made. A lack of transparency also raises concerns about accountability and the ability to clarify lending outcomes to customers or regulatory bodies.
5. Errors: Over-reliance on AI without appropriate human oversight can lead to errors or unintended consequences. Human intervention is crucial for reviewing and validating lending decisions to ensure fairness, mitigate risks and maintain ethical practices.
The impact of AI on the lending industry is still unfolding. However, it is apparent that AI is having a significant impact on the way that lending is done, including making lending faster, more efficient, and more accurate which leads to better outcomes for both lenders and borrowers.
It is important to note that while AI brings numerous benefits to the financial lending industry, it also raises ethical and regulatory considerations, such as transparency, bias mitigation and data privacy. Regulatory frameworks are continuously evolving to address these challenges and ensure responsible AI adoption in lending practices.
AI is often seen as a magical technology that can solve any problem. However, AI is only as good as the hardware that supports it. Just like a penthouse cannot stand without a strong foundation, AI cannot function without powerful chips.
Many people do not understand this limitation of AI. They believe that AI is capable of anything, if the software is good enough. However, this is not the case. The hardware is just as important as the software, if not more so. For example, if you want to train a large language model, you will need a lot of computing power. This is because large language models require a lot of data to train, and the training process is very computationally intensive.
If you do not have enough computing power, the training process will be slow, and the model will not be as accurate. This is why it is important to have powerful chips when you are working with AI. The good news is that the hardware for AI is constantly improving. As chips become more powerful, AI will become more capable. This means that in the future, AI will be able to solve even more problems than it can today.
How Semiconductor Chips are Transforming AI
Semiconductor chips are at the heart of every AI system. They are used to process data, train models, and make predictions. As AI technology continues to develop, the demand for semiconductor chips is growing rapidly. Semiconductor chips are becoming increasingly powerful, which allows AI systems to process more data and train more complex models. Secondly, these chips are also becoming more efficient which allows AI systems to run on less power and generate less heat. This in turn will lower the usage of electricity and lower the cost of running the models making it more affordable for users.
Global growth of Semiconductor Chip is expected to grow at a CAGR of 8.8% from 2022 to 2028, reaching a value of $1.38 trillion by 2028.
GPUs are expected to grow at the fastest rate of all the types of semiconductor chips. This is because GPUs are becoming increasingly important for a wide range of applications, including artificial intelligence, ML, and gaming. The growth of the GPU market is being driven by the increasing demand for GPUs in AI and ML applications. GPUs are well-suited for these applications because they can perform complex calculations quickly and efficiently. GPUs are also being used in gaming applications, as they can provide high-quality graphics.
The growth of the GPU market is expected to continue in the coming years. As AI and ML applications become more widespread, the demand for GPUs is expected to increase. Additionally, the growth of the gaming industry is also expected to drive the demand for GPUs.
This boom in generative AI, characterised by innovative platforms such as ChatGPT and Bard AI, is not only capturing attention but also propelling substantial investments in the field of artificial intelligence. As these advanced AI systems demonstrate remarkable capabilities in generating human-like text and engaging in meaningful conversations, the demand for powerful computing resources has soared. Consequently, this renewed interest and recognition of the transformative potential of generative AI have reignited the excitement surrounding AI chips.
AI chips, also known as AI accelerators or neural processing units (NPUs), are specialised hardware components designed specifically to enhance the performance and efficiency of AI tasks. They are tailored to handle the computational requirements of complex neural networks, enabling faster and more efficient execution of AI algorithms.
While GPUs, or Graphics Processing Units, are specialised hardware components originally designed for rendering images and graphics in computer games and visual effects. However, their parallel processing capabilities and high computational power have made them increasingly valuable for a wide range of applications, including AI and Generative AI. GPUs also excel at performing many calculations simultaneously, making them well-suited for the computational demands of AI and ML algorithms. Traditional central processing units (CPUs) are designed for sequential processing, executing one instruction at a time, while GPUs can handle thousands of parallel tasks concurrently. This parallelism enables GPUs to significantly accelerate AI workloads, leading to faster training and inference times. The increased investment and focus on AI chips are driving a wave of innovation, with companies vying to develop cutting-edge solutions to meet the evolving demands of generative AI. The competition among chip manufacturers and technology giants is leading to advancements in architecture, memory systems, and specialized circuits, all aimed at pushing the boundaries of AI performance.
Leading chipmaker performance in key AI categories
Source: Company self-disclosure
Based on data collected from PitchBook VC deal for AI startups achieve a good outcome due to M&A activities. With the limited VC funding, this encourage more M&A activities for startups that align with product that requires incumbents. This is due to market cycles similar to the rest of the technology sector. 2021 was a boom year for chip design companies and many of them managed to raise VC mega-deals. In 2022, the market took a turn due to the economic slowdown and geopolitics of US-China on-chip fabrication. Subsequently, this tension and recent policy change of US export controls, the AI semiconductor market has split into 2 mainly those in China and outside of China.
AI and ML semiconductor VC deal
Source: PitchBook
AI and ML semiconductor VS deals value ($)
Source: PitchBook
Another key driver that is driving VC deals in this sector is inference-based architectures. AI chips can be split into two categories: modelling and inferencing. The two main types of semiconductors used in AI system are modeling chips and inferencing chips. So, what are the difference between these two chips. Modeling chips are used to train AI models while inferencing chips are used to make predictions. Modeling chips are typically more powerful and very expensive while inferencing chips are usually less powerful and less expensive. Modeling focused startups set benchmarks based on the time required to train and calculate the efficiency of common benchmark models. These startups would be competing with Nvidia GPUs. While inferencing startups will be comparing their speed and efficiency of inference on standard models, however, they are primarily competing on energy efficiency of comparable performance
Source: PitchBook
Conclusion
Semiconductor chips are the backbone of AI, propelling their development and deployment. As hardware is just as important as software in enabling the full potential of AI. Powerful chips are essential for training large models and processing vast amounts of data accurately and efficiently. The growth of the semiconductor chip market is driven by the increasing demand for GPUs, which are vital in AI, ML, and gaming applications. GPUs excel at complex calculations and parallel processing, making them ideal for AI workloads.
Moreover, the rise of generative AI, exemplified by innovative platforms like Chat GPT and Bard AI, has sparked significant investments in AI chips. AI accelerators or neural processing units (NPUs) are specialised hardware designed to enhance AI performance and efficiency, while GPUs, originally for graphics, have found extensive use in AI due to their parallel processing capabilities. The AI ship market is expected to see significant growth, unlocking the power of AI in molding the future.
What Is the Internet of Things (IoT)?
IoT is the all-encompassing term for the aggregate collection of network-enabled devices, excluding traditional computers like laptops and servers. Types of network connections can include Wi-Fi connections. Artificial intelligence (AI) deals with the creation of systems that can learn to emulate human tasks using their prior experience and without any manual intervention. IoT can also make use of AI and generative AI to aid in making data collecting processes easier and more dynamic. The benefits to IoT systems to organisations varies in benefits from industry specific, and some can be applicable across multiple industries.
Below on Figure 1 is an example of the process breakdown for an IoT system, which can be broken down to 3 main phases, (i) collecting data, (ii) collect and transfer data and (iii) analyse data and act.
Data is essential to these IoT systems, for which companies that implement these systems consider data as a business moat and provides a key advantage for the business. As the data that is collected improves the existing AI system which can then enable users or IoT systems to quickly generate new content bases on inputs, this is an example of what is called generative AI. Generative AI enables users of the data to quickly generate new content based on inputs, whether that data is a single input (unimodal input) or multiple data inputs (multimodal). These data inputs are usually connected via an IoT device, examples such as a sensor, antenna or microcontroller.
AI-based safety technologies (i.e., IoT) helps business with the following (i) reduce risk across operations, (ii) increases efficiency for digital workflows for frontline workers and (iii) allows the physical platforms to integrate with the data to drive higher asset utilisation in the field.
Real world applications have shown there is a used case for the system integrating with IoT devices (data collector), its cloud operator (data storage) and generative AI (data processing and forecast new data). A real-life example is an American Oilfield service provider (Liberty Energy), using Samsara’s (IoT solutions provider) Video based safety for proactive driver coaching when operating within the parameters of the work field. Within the work field there are cameras (IoT device) which feed the video (data) to the AI programme (generative AI) and gives the driver an informed decision to tell if there are any obstruction to the driver, leading to less human error and increase efficiency.
Another example and used case for IoT and Generative AI is a UK company Lanes which is leader in wastewater utility solutions provider and the largest independent drainage specialist in the UK. Lanes shared that using Samsara helped to reduce fuel consumption by improving driving behaviour for their entire fleet. This allowed them to reduce overall carbon emissions even as their fleet continues to grow. It’s also worth mentioning that lanes adopted Samsara’s Video Based Safety and have decreased the frequency and severity of accidents. They lowered their insurance premiums and reduced the cost of each claim, generating an annual insurance savings of GBP 250,000 per year.
This type of digital transformation, IoT and generative AI, for physical operations is becoming strongly adopted in diverse industries such as construction, field services, manufacturing and retail which are the backbone of the global economy.
Competitive advantage for Businesses:
- Data is essentially a moat for these companies and are a key advantage for the business.
- AI-based safety technologies (i.e., IoT) help (i) reduce risk across operations, (ii)increases efficiency for digital workflows for frontline workers and the platforms are integrated with data to drive higher asset utilisation in the field.
- Customer example, Samsara uses their proprietary programme in the case of vehicle tracking and maintenance, for AI powered Video Based Safety application to improve workplace safety, reduce insurance premiums and lower corporate risk.
- Cost saving and improves ESG outfit to companies that use their platform alongside AI for safety checks.
- Companies in this space can be gross profitable level. Our durable and increasingly efficient growth demonstrates the large and growing opportunity for digital transformation across the world of physical operations.
- Generative AI helps reduce time and money spend on manpower. With alert notification being used in safety product, i.e. hours or hundreds of hours of video footage are saved.
Risks. There are mainly two major concerns for IoT devices, and they are Privacy and Security.
- IoT Privacy. Companies that manufacture and distribute IoT devices usually asks the user to input their personal information, which poses a risk to the user or company using the device, as the information can be taken.
- IoT Security. It usually takes only 1 unsecured device to hack into the whole network and render the whole system useless or leading either to invalid/false data being recorded.
Revenue projections for Generative AI and its impact to IoT.
Certain conclusions can be drawn from the use of generative AI and IoT in which the technology is corelated and complement each other to form processes that help in real life applications. With this theory of co-dependency, we can deduce that as there is more revenue towards Generative AI there will also be a similar growth towards IoT technology. Below is the forecasted future revenue for Generative AI across many industries.
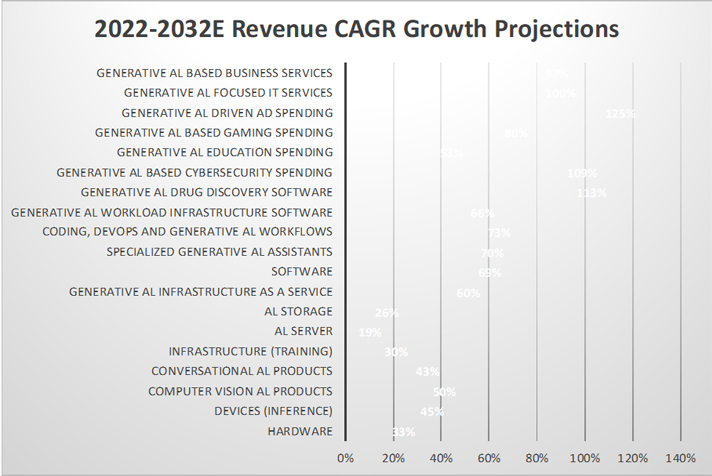
Figure 3: 2022-2032E Revenue CAGR Growth Projections (Source: Bloomberg, IDC, eMarketer, Statista)
Figure 4: Generative AI Revenue Projections (Source: Bloomberg, IDC, eMarketer, Statista)
The impact of generative AI and IoT are co-dependent on each other’s developments and are strongly linked, with not only the device but the data that is recorded, stored, used and developed. The industry has its competitive advantages and uses in business as well as its risks associated, however the conclusion is there is a stronger case for the use case outweighing the risk.
100Soonicorns powered by ScaleUp Accelerator Sdn Bhd (ScaleUp Malaysia) and Proficeo Consultants Sdn Bhd (Proficeo) in partnership with Penjana Kapital Sdn Bhd (Penjana Kapital) and Malaysia Digital Economy Corporation (MDEC) will kick off the new Cohort in June 2023.
Coined from the phrase “soon-to-be unicorns”, Soonicorns are startups with the growth potential to become Unicorns. The selected Founders are all Chief Executive Officers or C-Level Founders. To qualify, startups need to have raised at least US$1 million from a Venture Capital firm or institutional investor; or have generated US$2 million in revenue over the last 12 months.
The 100Soonicorns programme not only offers invaluable peer coaching and mentorship, but also creates a unique space for the selected Founders to connect and build relationships with each other. This sense of community and shared experience is the Unique Selling Proposition (USP) of the programme.
You will also get to learn from Unicorn founders who share their experience in building a Unicorn as well as the challenges they faced taking their company from startup to Unicorn. Unicorn founders who have shared include Moses Lo, the CEO of Xendit Ltd and Steve Melhuish the Co-Founder and former CEO of PropertyGuru.
To date, the programme has onboarded 24 Soonicorns, including 5 companies that have been funded by Penjana VCs like Ablr, Accendo Technologies, Easybook, Naluri and RPG Commerce.
The participating Founders of the first 2 cohorts are:
1. Amanda Chin, CEO, Ablr Malaysia
2. Sharma Lachu, CEO & Founder, Accendo Technologies Sdn Bhd
3. Ramachandran Muniandy, CEO & Co-Founder, Asia Mobility Technologies Sdn Bhd
4. Giden Lim, CEO & Co-Founder, BLOOMTHIS Flora Sdn Bhd
5. Benjamin Croc, CEO, Briohr Sdn Bhd
6. Ang Xing Xian, CEO, CapBay
7. Sharala Devi Balakrishnan, CEO, Center of Applied Data Science (CADS)
8. Kuna Kathigesan, Group CEO, Commerce DotAsia Ventures Sdn Bhd
9. Lee William, MD, Easybook (M) Sdn Bhd
10. Clarence Leong, CEO & Founder, EasyParcel Sdn Bhd
11. Suthan Mookaiah, CEO, GASSTN Sdn Bhd
12. Muhamad Nasir Habizar, CEO & Founder, Govicle Sdn Bhd
13. Effon Khoo, CEO & Founder, Kakitangan.com
14. Nadira Yusof, CEO & Founder, Kiddocare Sdn Bhd
15. Tunku Danny Nasaifuddin Mudzaffar, CEO & Founder, Microleap PLT
16. Azran Osman Rani, CEO, Naluri Hidup Sdn BHd
17. Swee Wai Hoow, CEO, Newswav Sdn Bhd
18. Keong Chun Chieh, CEO, Ominent Sdn Bhd
19. Dr. Kev Lim, CEO, Qmed Asia (QueueMed Healthtech Sdn Bhd)
20. Melvin Chee, CEO, RPG Commerce Sdn Bhd
21. Parthiven Shanmugan, CEO, TixCarte Sdn Bhd
22. Kendrick Tan, COO & Co-Founder, Virtualtech Frontier Sdn Bhd
23. Ooi Boon Sheng, CEO, Web Bytes Sdn Bhd
24. Low Ziwei, Director & Co-Founder, Zcova Sdn Bhd
Are you a high-growth startup looking to become Malaysia’s next Unicorn? Do you want to learn from the best and build relationships with top 100 startup founders in the country?
“100Soonicorns” is the first C-Level peer coaching programme for tech-only startups, designed by Proficeo and ScaleUp Malaysia and in partnership with Penjana Kapital and MDEC. Meet and learn from regional and global Unicorn founders and Investors who can support your next Series A/B raise. Application now open for the next cohort at www.proficeo.com.
DISCLOSURES AND DISCLAIMER |
This Newsletter is strictly informational and is issued Penjana Kapital Sdn Bhd (“PKSB”) on the basis that it is only for the information of the particular person to whom it was provided. This document may not be copied, reproduced, distributed or published by any recipient for any purpose unless Penjana Kapital Sdn Bhd’s prior written consent is obtained. This newsletter has been prepared for information purposes only and is not intended as an offer to sell or a solicitation to buy any securities, and/or any other product in Public or Private markets. Penjana Kapital Sdn Bhd is not making any recommendation to buy any securities or other product and the information provided should not be taken as investment advice.
It has been prepared without regard to the individual financial circumstances and objectives of persons who receive it. Penjana Kapital Sdn Bhd has no obligation to update its opinion or the information in this newsletter and Penjana Kapital Sdn Bhd recommends that you independently evaluate particular investments and strategies and seek the advice of a financial adviser prior to entering into any transaction. The appropriateness of a particular investment or strategy will depend on your individual circumstances and objectives. The information herein was obtained or derived from sources that Penjana Kapital Sdn Bhd believes are reliable, but while all reasonable care has been taken to ensure that stated facts are accurate and opinions fair and reasonable, we do not represent that it is accurate or complete and it should not be relied upon as such. All opinions and estimates included in this newsletter constitute our views as of this date and are subject to change without notice.
Penjana Kapital Sdn Bhd is not acting as your advisor and does not owe any fiduciary duties to you in connection with this newsletter and no reliance may be placed on Penjana Kapital Sdn Bhd for advice or recommendations of any sort. Nothing in this newsletter shall constitute legal, accounting or tax advice, or a representation that any transaction or investment is appropriate for you taking into account your investment objectives, financial situation and particular needs, or otherwise constitutes any such advice to you. Penjana Kapital Sdn Bhd makes no representations or warranties, express or implied, with respect to the accuracy of the information or fitness for any particular purpose and does not accept any liability (including but not limited to any direct, indirect or consequential losses, loss of profits and damages) for any use you or your advisors make of the contents of this newsletter or for any loss that may arise from the use of this newsletter or reliance by any person upon such information or opinions provided in the newsletter. This newsletter has been prepared by the analysts of Penjana Kapital Sdn Bhd. Facts and views presented in this newsletter may not reflect the views of or information known to other business units within Penjana Kapital Sdn Bhd. This information herein is not intended to constitute “research” as it is defined by applicable laws. This newsletter is not directed to or intended for distribution to or use by any person or entity who is a citizen or resident of or located in any locality, state, country or other jurisdiction where such distribution, publication, availability or use would be contrary to law or regulation. The information provided in this document has been obtained or derived from sources believed to be reliable. Penjana Kapital Sdn Bhd does not guarantee its accuracy or completeness and does not assume any liability for any loss that may result from the reliance by any person upon any such information or opinion. Such information or opinions are subject to change without notice, are for general information only and is not intended as an offer to sell or a recommendation/ solicitation to buy any securities, foreign exchange or other product.